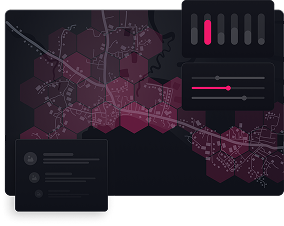
Demand Forecasting Solution for Transportation Services
Urban transportation logistics often face inefficient vehicle placement and fluctuating demand. To address these issues, we developed a predictive demand model to tackle the challenges of urban transportation logistics. Using historical data, the solution helps transport companies to strategically position vehicles across city zones, ensuring optimal resource allocation and accurately meeting customer demand.
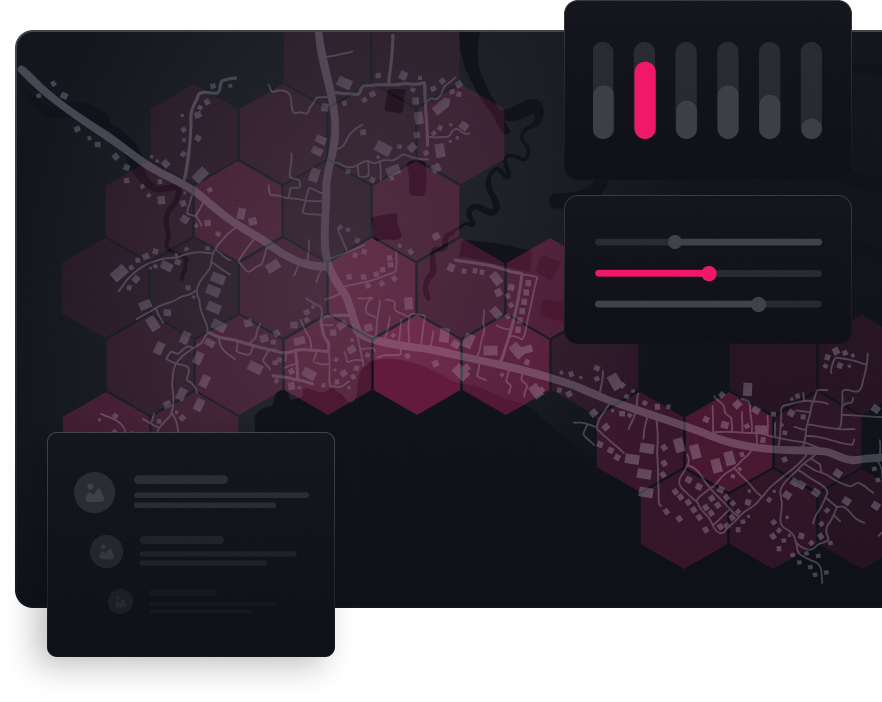
Solution
The predictive model significantly improves operational efficiency for transport companies by increasing order volumes and minimizing vehicle idle times. The model incorporates key factors, including:
Seasonality
National Holidays
Weather Conditions
Tech Stack
The project was built with a powerful blend of methodologies and tools, including:
Geospatial data processing to analyze urban mobility patterns
Autoregressive and Random forest regression models for predictive accuracy
Third-party API for real-time weather data
Key technologies used for development and data management included:
Python
Scikit Learn
PostgreSQL
Results
The implementation of this system delivered measurable improvements across key operational metrics:
-
Higher profitability through increased service orders and reduced downtime
-
Optimized vehicle distribution based on precise demand forecasts
-
Streamlined operations, minimizing wasted resources and enhancing service reliability
By blending advanced machine learning, geospatial analysis, and real-time data integration, this solution improved daily operations and allowed transport companies to respond to fluctuating demand. This solution set a new benchmark for efficiency in the transportation industry, delivering value to businesses and their customers.
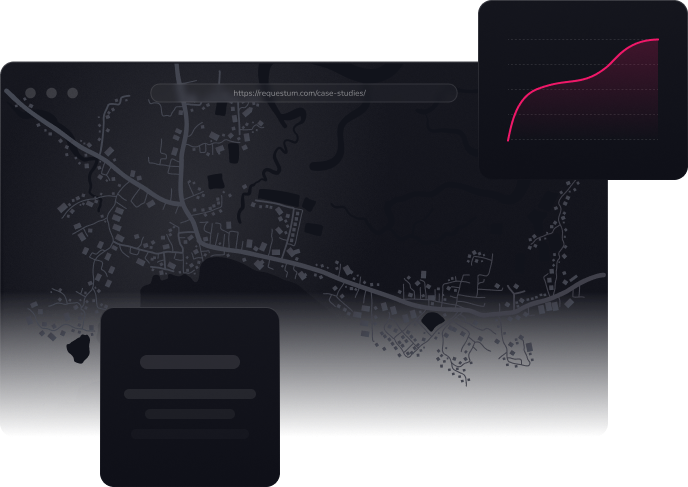